Red-Blue Teaming Affairs
Release time:2024/01/25
Back list
With the widespread application of AIGC large models in multiple fields, the importance of their attack and defense strategies is becoming increasingly evident. The complexity of these models provides new vulnerabilities and challenges for attackers, and the rapid advancement of technology means that attack methods are constantly evolving and upgrading. Public concern about privacy and data security, coupled with increased legal and ethical requirements for AI systems, makes ensuring the security and reliability of large models even more critical.
To achieve this, it is necessary to continuously improve attack and defense strategies, integrate security considerations into model design, and ensure that these models not only provide efficient services but also comply with ethical standards and legal regulations in society through interdisciplinary cooperation.
"Red-Blue Teaming" has become an important means for leading technology giants like OpenAI to assess the reliability and robustness of AIGC models.
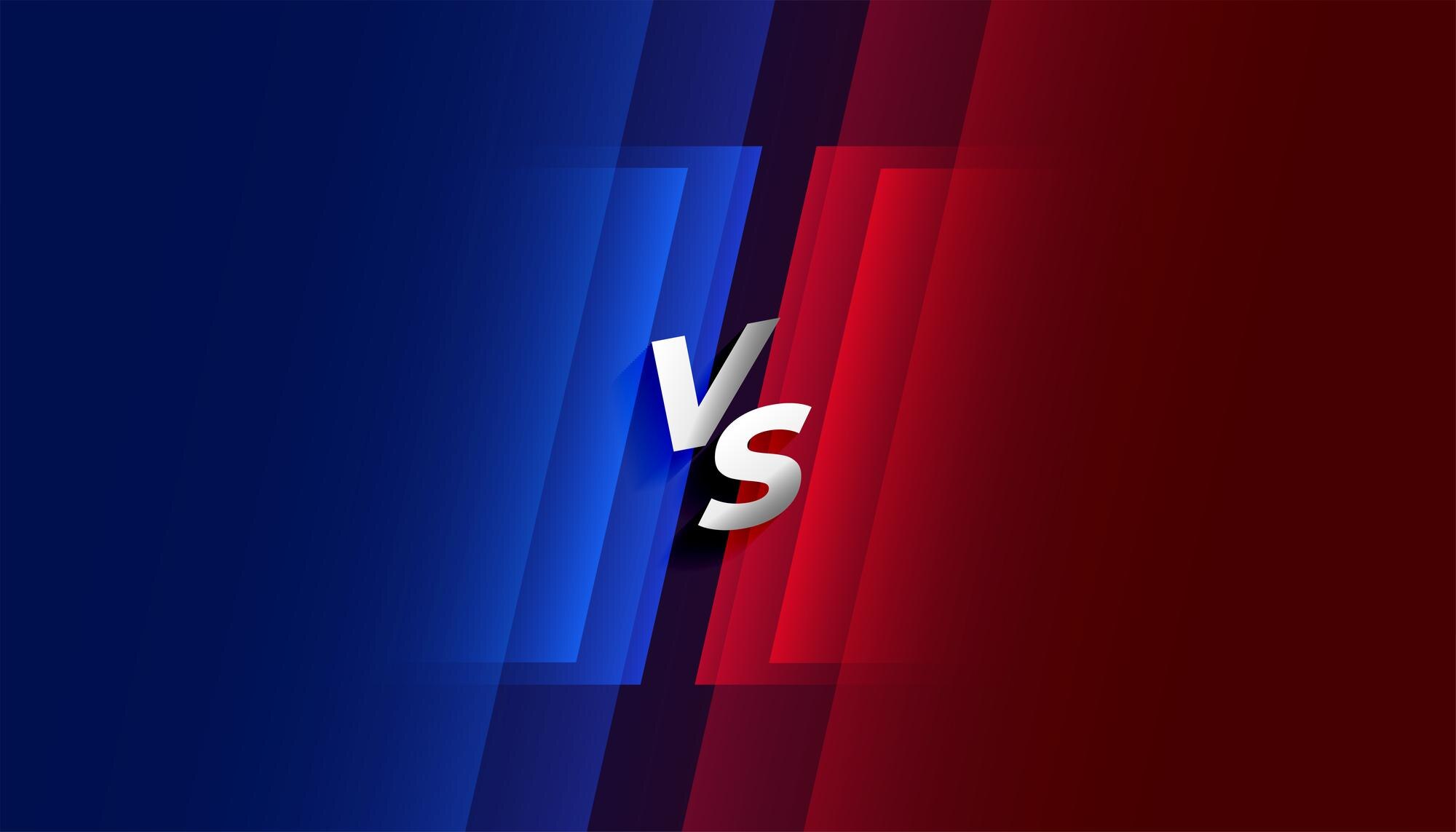
Why Red-Blue Teaming
Through continuous testing and challenging of models (the role of the red team), vulnerabilities or weaknesses in the model can be discovered and fixed. This is crucial for preventing malicious exploitation of the model or unpredictable behavior in complex scenarios. The challenges from the red team can expose the model's shortcomings when dealing with complex, ambiguous, or misleading inputs. Subsequently, the blue team works to improve the model, enhancing the quality and accuracy of its generated content.
Artificial intelligence models can unintentionally learn and replicate biases present in their training data. Red-Blue Teaming helps identify and reduce these biases, ensuring fair and non-discriminatory model outputs. As technology evolves and attacker strategies change, models face evolving security threats. Red-Blue Teaming allows models to adapt to these changes, maintaining the effectiveness of their defense mechanisms. By demonstrating that models can effectively handle various challenges and attacks, Red-Blue Teaming helps build user trust in artificial intelligence systems.
As ethical and legal regulations on artificial intelligence continue to strengthen, conducting Red-Blue Teaming can help ensure that models comply with relevant ethical standards and legal requirements, especially when dealing with sensitive information and decision-making. The process of Red-Blue Teaming itself serves as a catalyst for technological innovation, driving developers to constantly seek new methods and technologies to improve model performance and security. Therefore, Red-Blue Teaming is crucial for ensuring the safety, reliability, fairness, and ethical operation of AIGC large models and also contributes to the advancement and development of artificial intelligence technology.
Principles of Red-Blue Teaming
• Red Team (Attacker): The red team's task is to challenge and test large models, discovering their weaknesses. This includes generating content that violates guidelines, causing the model to make incorrect judgments or responses, or attempting to deceive the model into generating inappropriate content. The red team may employ various strategies such as:
○ Asking misleading or ambiguous questions.
○ Trying to guide the model to generate biased, discriminatory, or inaccurate responses.
○ Using complex or unclear language to attempt to make the model generate errors.
• Blue Team (Defense): The blue team's task is to protect and strengthen the model against the red team's attacks. This typically involves ongoing model training and adjustments, as well as the formulation of stricter policies and guidelines to reduce the risk of the model generating inappropriate content. The blue team's work includes:
○ Improving the model's filtering and monitoring mechanisms.
○ Regularly updating the model to address newly emerging attack methods.
○ Stress-testing the model to ensure its stability and reliability in various situations.
The Red-Blue Teaming process is a continuous cycle. The red team continues to look for new attack methods, while the blue team continually strengthens the model's defenses. Through this process, the model gradually improves its ability to combat the generation of inappropriate content while maintaining accuracy and appropriateness in its responses. This is crucial for developing high-quality, secure artificial intelligence models.
Red-Blue Teaming Examples
• Red Team Attack Scenarios:
○ Adversarial Attacks: Using adversarial samples to deceive the model. For example, making tiny but precise modifications to input data that are almost imperceptible to humans but can lead to incorrect predictions or classifications by the model.
○ Data Poisoning: Deliberately injecting incorrect information or biased data into the training data, causing the trained model to inherit these biases or errors.
○ Model Reverse Engineering: Attempting to understand the internal workings of the model to discover exploitable weaknesses.
• Blue Team Defense Strategies:
○ Data Cleaning and Validation: Ensuring the quality of training data, removing biased or erroneous data, and using validation techniques to ensure the quality of input data.
○ Adversarial Training: Including adversarial samples in the training process to enable the model to recognize and handle these samples correctly.
○ Model Regularization: Applying regularization techniques to reduce the model's sensitivity to noisy data.
○ Monitoring and Logging: Real-time monitoring of the model's outputs, recording and analyzing abnormal behavior to respond quickly to potential security threats.
The core of these examples and methods is continuous testing, evaluation, and adjustment to ensure the model's stability and security in various situations. Importantly, Red-Blue Teaming is not a one-time activity but an ongoing process that needs continuous updating and improvement as technology evolves and attack methods change.
Collaborating with DataOceanAI on Red-Blue Teaming
DataOceanAI, as a globally renowned brand, focuses on providing high-quality training data for deep learning models to enhance their accuracy and performance.
In the field of AIGC, DataOceanAI plays a crucial role. On one hand, they possess a vast amount of professionally gathered, recorded, and annotated de-identified data. These datasets lay the foundation for training reliable and trustworthy large-scale AI models. On the other hand, DataOceanAI has professional annotators who can act as human trainers in Red-Blue Confrontation for large model training. They guide these large models to err, identify their weak points, and ultimately enhance the models' robustness through optimized training data, thereby promoting the development and implementation of more accurate and trustworthy large-scale models.
DataOceanAI can customize AI training teams according to specific standards, making the Red-Blue Confrontation process both efficient and effective. This is particularly important for companies pursuing unbiased, error-free generative AI models. As technology evolves, the role of Red-Blue Confrontation in AI development becomes increasingly crucial. Additionally, DataOceanAI provides a plethora of deceptive images and voice data to attack weak recognition systems, while also offering comprehensive anti-deception algorithm strategies, data watermarking, and other features to protect user privacy and enhance the robustness of existing large models.